#encoding=utf-8
from tkinter import *
from tkinter.colorchooser import *
from tkinter.filedialog import *
class Application(Frame):
def __init__(self, master = None):
super().__init__(master)
self.master = master
self.pack()
self.createWidget()
def createWidget(self):
# 创建主菜单栏
menubar = Menu(root)
# 创建子菜单
menuFile = Menu(menubar)
menuEdit = Menu(menubar)
menuHelp = Menu(menubar)
# 将子菜单加入到主菜单栏
menubar.add_cascade(label='文件(F)', menu=menuFile)
menubar.add_cascade(label='编辑(E)', menu=menuEdit)
menubar.add_cascade(label='帮助(H)', menu=menuHelp)
# 添加菜单项
menuFile.add_command(label='新建', accelerator='ctrl + n', command=self.test)
menuFile.add_command(label='打开', accelerator='ctrl + o', command=self.test)
menuFile.add_command(label='保存', accelerator='ctrl + s', command=self.test)
menuFile.add_separator() # 添加分割线
menuFile.add_command(label='退出', accelerator='ctrl + q', command=self.test)
# 将主菜单栏加到跟窗口
root['menu'] = menubar
# 创建上下文菜单
menubar2 = Menu(root)
menubar2.add_command(label='颜色', command = self.openAskColor)
menuedit = Menu(menubar2, tearoff = 0)
menuedit.add_command(label='剪切')
menuedit.add_command(label='复制')
menuedit.add_command(label='粘贴')
menubar2.add_cascade(label='编辑', menu=menuedit)
# 编辑区
w1 = Text(root, width=50, height=30)
w1.pack()
w1.bind('<Button-3>', text)
def test(self):
pass
def openAskColor(self):
s1 = askcolor(color='red', title='选择背景色')
root.config(bg=s1[1])
def test1(event):
# 菜单在鼠标右键单击的坐标处显示
menubar2.post(event.x_root, event.y_root)
if __name__ == "__main__":
root = Tk()
root.title('my window');root.geometry('450x300')
app = Application(master = root)
root.mainloop()
老师:问题很多,视频七分钟,百度两小时
如图,菜单栏虚线可以点击,并且点击后新弹出个窗口
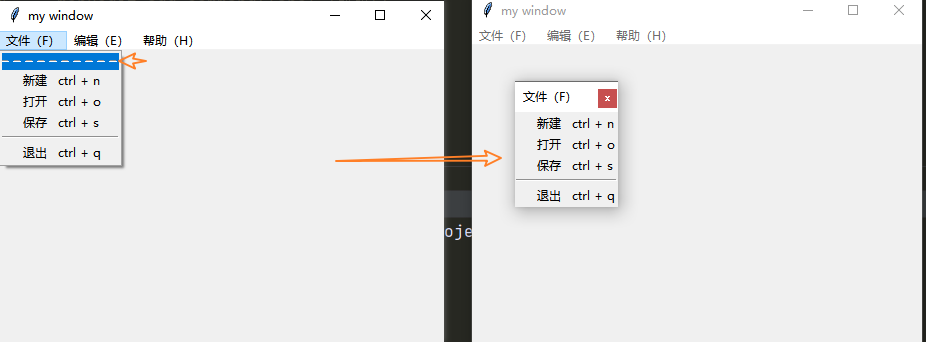
2.这些地方是调用组件么。组件第一个字母不都是大写
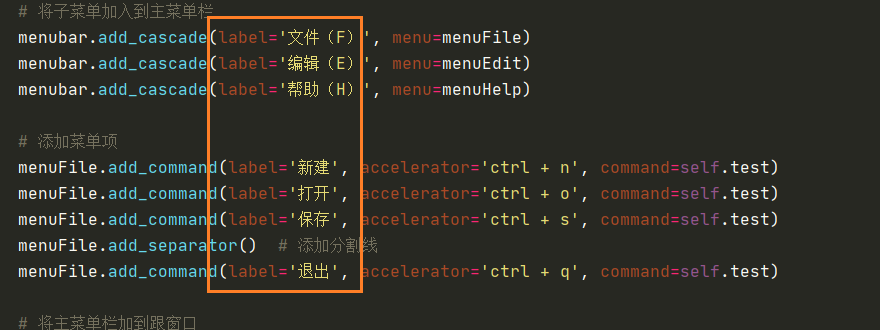
3.我这里总是显示这样的,不知道为什么
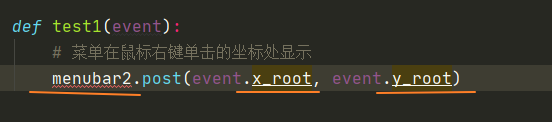